Details
- Identification
- ISSN: 1977-5296, DOI: 10.3011/ESARDA.IJNSNP.2021.1
- Publication date
- 1 June 2021
- Author
- Joint Research Centre
Description
Volume: 62, June 2021, pages 2-12,
Authors: Erik Branger, Zsolt Elter, Sophie Grape, Markus Preston
Uppsala University
Abstract:
Safeguards verification of spent nuclear fuel assemblies is frequently done by per forming non-destructive measurements, which are used to verify the completeness and correctness of operator declarations such as initial enrichment (IE), burnup (BU) and cooling time (CT) of the fuel. However, different irradiation histories may result in the same combination of CT, BU and IE, and such fuels may behave differently despite identically declared values. The goal of this work is to investigate what effect the irradiation history has on the ability to predict the fuel parameters using random forest regression. Random forest regression models were trained to predict the fuel parameters IE, BU and CT based on combinations of radiation signatures calculated from a previously modelled Pressurised Water Reactor (PWR) spent nuclear fuel library. The radiation signatures studied were the relative gamma-ray activities of Cs137, Cs134 and Eu154, their total gamma-ray activity, the total neutron emission rate and the parametrised early die-away time τ from the Differential Die-away Self Interrogation (DDSI) instrument. The performance of the models were tested on simulations of 2192 PWR fuel assemblies from the Ringhals 3 and 4 nuclear power plants in Sweden, which were simulated based on their documented irradiation histories. Despite significant differences in irradiation history between the training and testing data sets, the Ringhals assembly parameters could be predicted with similar accuracy as for assemblies in the training set. The relative gamma-ray activities were sufficient to predict the CT with an RMSE of 2 years, and adding a total gamma or total neutron signature allowed the BU to be predicted with an RMSE of 1.4 MWd/kgU. The DDSI early die-away time τ enabled an accurate IE prediction, with an RMSE of 0.16 w%. The differences between irradiation histories introduced a systematic bias where CT was overestimated by about 1 year and the BU by about 1.5 MWd/kgU.
Keywords: Nuclear safeguards, fuel parameter prediction, machine learning, random forest regression, irradiation history
Reference guideline:
Branger, E., Elter, Z., Grape, S., & Preston, M. (2021). Investigating the sensitivity to irradiation history when predicting fuel parameters using random forest regression. ESARDA Bulletin - The International Journal of Nuclear Safeguards and Non-proliferation, 62, 2-12. https://doi.org/10.3011/ESARDA.IJNSNP.2021.1
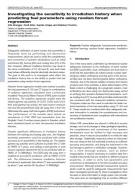