Details
- Identification
- ISSN: 1977-5296, DOI: 10.3011/ESARDA.IJNSNP.2021.7
- Publication date
- 1 December 2021
- Author
- Joint Research Centre
Description
Volume: 63, December 2021, pages 3-14,
Special Issue on Data Analytics for Safeguards and Non-Proliferation
Authors: Riccardo Rossa and Alessandro Borella
SCK CEN Belgian Nuclear Research Centre
Abstract:The verification of spent nuclear fuel is a major task during a safeguards inspection and inspectors have to verify both the correctness and completeness of the operator declaration. The traditional way to verify spent fuel is with non-destructive assays (NDA) relying on the radiation emission from the fuel. The NDA measurement results are then compared with estimates based on operator declaration of initial enrichment, burnup, and cooling time. However, the radiation emission from spent fuel is affected by the fuel parameters and irradiation history, and research is ongoing to improve the data analysis of NDA measurement results. In this work artificial neural networks were developed to infer the initial enrichment, burnup, and cooling time of spent fuel assemblies from simulated NDA measurements with the Forkball instrument. Several neural networks architectures and detector responses were compared to find the optimal network configuration to infer the spent fuel parameters. Results show that the cooling time is the most challenging parameter to estimate and the associated data processing step plays a crucial role in its reliable estimate. The combination of multiple detector responses also leads to a significant improvement in the determination of the initial enrichment, burnup, and cooling time. The optimal neural networks in this study are able to determine the initial enrichment and burnup within 12%, and the cooling time, using the data processing step, within 4%.
Keywords: neural networks; machine learning; spent fuel; NDA; initial enrichment; burnup; cooling time
Reference guideline:
Rossa, R., & Borella, A. (2021). Inferring initial enrichment, burnup, and cooling time of spent fuel assemblies using artificial neural networks.
ESARDA Bulletin - The International Journal of Nuclear Safeguards and Non-proliferation, 63, 3-14.
https://doi.org/10.3011/ESARDA.IJNSNP.2021.7
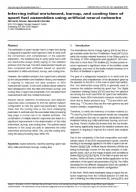